Table of contents
- Introduction
- Printers
- Methods & Materials
- Case Studies
- Visualisations
- Reflection
- Conclusion
- Division of Labour and Reflection on Learning
- References
Reflection
Besides the biases that were mentioned under the “Material and methods” section, which are due to the use of the ECCO database, there are some that also come from the approaches or methodologies that we utilised. (I.e. as we only looked at DIs, we might have missed some leads due to that).
Missing DIs
One obstacle that made it at times hard to find leads and possibly ended up misleading us, were the DIs missing from our clusters. After starting the analysis phase of the project, it became apparent that not all of the DIs that could be of interest to us ended up in our final set of clusters. Such was the case for example with one of the Bowyer DIs that appeared in one of the first books analysed after our second annotation round.
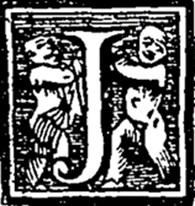
After discovering the DI shown above was not in our clusters, it was quickly acknowledged that e.g. “the_man_and_woman” (the variations included) and the “winged_woman_and_old_man” were also not discoverable through our data. Instead, they could be found through looking at the contents (in this case the books’ decorations) in the Compositor of the same books that had some DIs that were in our clusters. There was additionally a case where a DI appeared in our clusters during the first round of annotation, but as it did not match with any of the other DIs in its initial cluster, it was not marked down as a pattern by the annotator. Although it matches with the DIs in our cluster of “Bowyer_A_two_people_facing_to_their_right”, because it was a lot brighter than the DIs there, the model placed it in another cluster. As the separate clusters were annotated by different annotators, this matching but brighter DI was left outside of our final clusters.
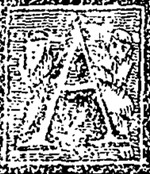
As demonstrated, the use of Compositor besides our own data ended up being crucial, as it offered us a user interface where the book decorations could be perused at the same time in big quantities. On the other hand, there were also instances where Compositor was missing a DI which could be found in our data. Such was the case with Poems on several occasions. Overall, our database and the Compositor complemented each other well, and using them together meant that we did not have to rely only on our clusters of the database. However, the missing DIs ultimately affected the connections we were able to make and the completeness of our visualisations. The decorative initials missing from our clusters may have led us to overlook book titles that contain initials from DI sets we are interested in. This means we are not able to systematically connect all related book titles with Richardson, or to draw more meaningful conclusions from them.
Missing Genre Classification
In reflecting on the data, it is evident that 16.20% of the module data remains incomplete, with The Natural History of Carolina, Florida, and the Bahama Islands being a notable example. This missing information introduces a significant bias, as the absence of such critical data limits our ability to fully analyse and classify the decorative initials within their respective genres. If we can better define the genre or module for these incomplete datasets, our analysis will become more comprehensive, enabling us to link decorative initials more accurately to their respective ECCO book genres.
Challenges in Identifying and Harmonizing Historical Printers
Harmonising printers across historical records is complicated by inconsistent naming conventions and limited concrete evidence. A notable example involves printers “Bowyer, W.” and “B., W.,” who appear to have used the same decorative initials in their printed works. This stylistic similarity suggests a possible identity overlap, yet the absence of concrete proof means we cannot confirm unequivocally whether they are the same family, or company. This problem is further compounded when printers used initials, pseudonyms, or alternative spellings to either protect their identity or differentiate editions. Additionally, printers could collaborate, use shared equipment, or change locations frequently, leaving behind records that are difficult to map directly.
Ideas for Improvement and Future Research
With focused effort on DIs rather than DIs and factotums, our cluster cleaning project would have led to a more complete collection of the DIs we ultimately focused on. As we started the project by annotating both decorative initial and factotum clusters, we lost annotation effort that could have been focused on DIs. By focusing on the DIs from the start we could have used more than one person annotating each cluster. As the initial clusters were uneven in size and “noise”, we should have also put more value on “picking clusters clean” of any set of DIs for more complete collection. In hindsight, it is clear that every instance of a specific DI can be an important lead to a book it was used in, or to connect it with other initials belonging to a set used by a printer. In the case of the two similar “W” DIs presented earlier (See Richardson and the case of the Two DIs), manually finding more DIs from books that are connected by mutual decorative initials was time consuming, and was later replaced by cross-referencing with the master file containing all 50 000 DIs.
Furthermore, in this project we looked into only DIs but similar work could be done on the factotums which we decided to leave aside from the scope of our project. Not to mention that looking into possible combinations of HPs, DIs, and FTs could be another way to find leads for discovering new information. We believe that there could be a need for a more systematic way of clustering the DIs and FTs in the future, so it might be that a restructuring of the current system, and adding into it other elements of images besides FTs could be considered as the topic of an entire project. If that comes to fruition, then our present work in clustering the DIs and FTs, naming the DIs we had in the clusters of our project, and the biases and problems of the current DI and FT clustering system mentioned in this blog may be useful.